使用 OpenCV 和 Python 在直播中模糊人臉
本文將學習如何使用 OpenCV 和 Python 在直播中模糊人臉。這將是一個非常有趣的博客,讓我們開始吧!
我們最終結(jié)果的快照:
第 1 步:導(dǎo)入所需的庫
· 為圖像操作導(dǎo)入 cv2
· 為數(shù)組操作導(dǎo)入 Numpy
import cv2
import numpy as np
第 2 步:定義模糊函數(shù)
· 這里我們定義了 Blur 函數(shù)。
· 它需要 2 個參數(shù),圖像 img 和模糊因子 k 。
· 然后我們通過將高度和寬度除以模糊因子來簡單地計算內(nèi)核高度和內(nèi)核寬度。kw 和 kh 越小,模糊度越高。
· 然后我們檢查 kw 和 kh 是否為奇數(shù),如果它們是偶數(shù),則減 1 以使它們?yōu)槠鏀?shù)。
· 然后簡單地我們將高斯模糊應(yīng)用于我們的圖像并返回它。
def blur(img,k):
h,w = img.shape[:2]
kh,kw = h//k,w//k
if kh%2==0:
kh-=1
if kw%2==0:
kw-=1
img = cv2.GaussianBlur(img,ksize=(kh,kw),sigmaX=0)
return img
第 3 步:定義 pixelate_face 函數(shù)
· 這是一個簡單地為模糊圖像添加像素化效果的函數(shù)。
def pixelate_face(image, blocks=10):
# divide the input image into NxN blocks
(h, w) = image.shape[:2]
xSteps = np.linspace(0, w, blocks + 1, dtype="int")
ySteps = np.linspace(0, h, blocks + 1, dtype="int")
# loop over the blocks in both the x and y direction
for i in range(1, len(ySteps)):
for j in range(1, len(xSteps)):
# compute the starting and ending (x, y)-coordinates
# for the current block
startX = xSteps[j - 1]
startY = ySteps[i - 1]
endX = xSteps[j]
endY = ySteps[i]
# extract the ROI using NumPy array slicing, compute the
# mean of the ROI, and then draw a rectangle with the
# mean RGB values over the ROI in the original image
roi = image[startY:endY, startX:endX]
(B, G, R) = [int(x) for x in cv2.mean(roi)[:3]]
cv2.rectangle(image, (startX, startY), (endX, endY),
(B, G, R), -1)
# return the pixelated blurred image
return image
第 4 步:讓我們在實時提要中模糊面孔
· 下面的代碼是代碼的主要部分。
· 這里的 factor 定義了模糊量。
· 定義一個級聯(lián)分類器對象 face_cascade 來檢測人臉。
· 下載 haarcascade_frontalface_default.xml 文件
然后簡單地運行一個無限循環(huán),從網(wǎng)絡(luò)攝像頭讀取圖像,檢測其中的人臉,然后用像素化的人臉替換該人臉部分。
閱讀更多關(guān)于使用 HAARCASCADES 進行面部和眼睛檢測的信息
factor = 3
cap = cv2.VideoCapture(0)
face_cascade = cv2.CascadeClassifier('haarcascade_frontalface_default.xml')
while 1:
ret,frame = cap.read()
gray = cv2.cvtColor(frame,cv2.COLOR_BGR2GRAY)
faces = face_cascade.detectMultiScale(gray, 1.5, 5)
for (x,y,w,h) in faces:
frame[y:y+h,x:x+w] = pixelate_face(blur(frame[y:y+h,x:x+w],factor))
cv2.imshow('Live',frame)
if cv2.waitKey(1)==27:
break
cap.release()
cv2.destroyAllWindows()
讓我們看看完整代碼
import cv2
import numpy as np
def blur(img,k):
h,w = img.shape[:2]
kh,kw = h//k,w//k
if kh%2==0:
kh-=1
if kw%2==0:
kw-=1
img = cv2.GaussianBlur(img,ksize=(kh,kw),sigmaX=0)
return img
def pixelate_face(image, blocks=10):
# divide the input image into NxN blocks
(h, w) = image.shape[:2]
xSteps = np.linspace(0, w, blocks + 1, dtype="int")
ySteps = np.linspace(0, h, blocks + 1, dtype="int")
# loop over the blocks in both the x and y direction
for i in range(1, len(ySteps)):
for j in range(1, len(xSteps)):
# compute the starting and ending (x, y)-coordinates
# for the current block
startX = xSteps[j - 1]
startY = ySteps[i - 1]
endX = xSteps[j]
endY = ySteps[i]
# extract the ROI using NumPy array slicing, compute the
# mean of the ROI, and then draw a rectangle with the
# mean RGB values over the ROI in the original image
roi = image[startY:endY, startX:endX]
(B, G, R) = [int(x) for x in cv2.mean(roi)[:3]]
cv2.rectangle(image, (startX, startY), (endX, endY),
(B, G, R), -1)
# return the pixelated blurred image
return image
factor = 3
cap = cv2.VideoCapture(0)
face_cascade = cv2.CascadeClassifier('haarcascade_frontalface_default.xml')
while 1:
ret,frame = cap.read()
gray = cv2.cvtColor(frame,cv2.COLOR_BGR2GRAY)
faces = face_cascade.detectMultiScale(gray, 1.5, 5)
for (x,y,w,h) in faces:
frame[y:y+h,x:x+w] = pixelate_face(blur(frame[y:y+h,x:x+w],factor))
cv2.imshow('Live',frame)
if cv2.waitKey(1)==27:
break
cap.release()
cv2.destroyAllWindows()
這就是你在直播中模糊面孔的方式!
原文標題 : 使用 OpenCV 和 Python 在直播中模糊人臉
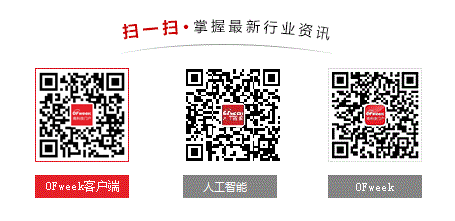
請輸入評論內(nèi)容...
請輸入評論/評論長度6~500個字
最新活動更多
推薦專題
- 1 UALink規(guī)范發(fā)布:挑戰(zhàn)英偉達AI統(tǒng)治的開始
- 2 北電數(shù)智主辦酒仙橋論壇,探索AI產(chǎn)業(yè)發(fā)展新路徑
- 3 “AI寒武紀”爆發(fā)至今,五類新物種登上歷史舞臺
- 4 降薪、加班、裁員三重暴擊,“AI四小龍”已折戟兩家
- 5 國產(chǎn)智駕迎戰(zhàn)特斯拉FSD,AI含量差幾何?
- 6 光計算迎來商業(yè)化突破,但落地仍需時間
- 7 東陽光:2024年扭虧、一季度凈利大增,液冷疊加具身智能打開成長空間
- 8 地平線自動駕駛方案解讀
- 9 封殺AI“照騙”,“淘寶們”終于不忍了?
- 10 優(yōu)必選:營收大增主靠小件,虧損繼續(xù)又逢關(guān)稅,能否乘機器人東風翻身?