如何使用Python將給定的圖像集進(jìn)行聚類?
<a onclick="parent.postMessage({'referent':'.kaggle.usercode.12234793.44545592.ShowMeWhatYouLearnt..featureMaps'}, '*')">featureMaps = <a onclick="parent.postMessage({'referent':'.kaggle.usercode.12234793.44545592.ShowMeWhatYouLearnt..model'}, '*')">model.predict(<a onclick="parent.postMessage({'referent':'.kaggle.usercode.12234793.44545592.ShowMeWhatYouLearnt..img'}, '*')">img)
## Plotting Features
for a onclick="parent.postMessage({'referent':'.kaggle.usercode.12234793.44545592.ShowMeWhatYouLearnt..maps'}, '*')">maps in <a onclick="parent.postMessage({'referent':'.kaggle.usercode.12234793.44545592.ShowMeWhatYouLearnt..featureMaps'}, '*')">featureMaps:
plt.<a onclick="parent.postMessage({'referent':'.matplotlib.pyplot.figure'}, '*')">figure(figsize=(20,20))
<a onclick="parent.postMessage({'referent':'.kaggle.usercode.12234793.44545592.ShowMeWhatYouLearnt..pltNum'}, '*')">pltNum = 1
for <a onclick="parent.postMessage({'referent':'.kaggle.usercode.12234793.44545592.ShowMeWhatYouLearnt..a(chǎn)'}, '*')">a in range(8):
for <a onclick="parent.postMessage({'referent':'.kaggle.usercode.12234793.44545592.ShowMeWhatYouLearnt..b'}, '*')">b in <a onclick="parent.postMessage({'referent':'.kaggle.usercode.12234793.44545592.ShowMeWhatYouLearnt..range'}, '*')">range(8):
plt.<a onclick="parent.postMessage({'referent':'.matplotlib.pyplot.subplot'}, '*')">subplot(8, 8, <a onclick="parent.postMessage({'referent':'.kaggle.usercode.12234793.44545592.ShowMeWhatYouLearnt..pltNum'}, '*')">pltNum)
plt.<a onclick="parent.postMessage({'referent':'.matplotlib.pyplot.imshow'}, '*')">imshow(<a onclick="parent.postMessage({'referent':'.kaggle.usercode.12234793.44545592.ShowMeWhatYouLearnt..maps'}, '*')">maps[: ,: ,<a onclick="parent.postMessage({'referent':'.kaggle.usercode.12234793.44545592.ShowMeWhatYouLearnt..pltNum'}, '*')">pltNum - 1], cmap='gray')
<a onclick="parent.postMessage({'referent':'.kaggle.usercode.12234793.44545592.ShowMeWhatYouLearnt..pltNum'}, '*')">pltNum += 1
plt.<a onclick="parent.postMessage({'referent':'.matplotlib.pyplot.show'}, '*')">show()
接下來我們將重點(diǎn)介紹如何來創(chuàng)建我們的聚類算法。設(shè)計(jì)圖像聚類算法在本節(jié)中,我們使用Kaggle上的 keep-babies-safe 數(shù)據(jù)集。https://www.kaggle.com/akash14/keep-babies-safe首先,我們創(chuàng)建一個(gè)圖像聚類模型,來將給定的圖像分為兩類,即玩具或消費(fèi)品,以下是來自該數(shù)據(jù)集的一些圖像。
以下代碼實(shí)現(xiàn)我們的聚類算法:##################### Making Essential Imports ############################
import sklearn
import os
import sys
import matplotlib.pyplot as plt
import cv2
import pytesseract
import numpy as np
import pandas as pd
import tensorflow as tf
conf = r'-- oem 2'
#####################################
# Defining a skeleton for our #
# DataFrame #
#####################################
DataFrame = {
'photo_name' : [],
'flattenPhoto' : [],
'text' : [],
}
#######################################################################################
# The Approach is to apply transfer learning hence using Resnet50 as my #
# pretrained model #
#######################################################################################
MyModel = tf.keras.models.Sequential()
MyModel.a(chǎn)dd(tf.keras.a(chǎn)pplications.ResNet50(
include_top = False, weights='imagenet', pooling='avg',
))
# freezing weights for 1st layer
MyModel.layers[0].trainable = False
### Now defining dataloading Function
def LoadDataAndDoEssentials(path, h, w):
img = cv2.imread(path)
DataFrame['text'].a(chǎn)ppend(pytesseract.image_to_string(img, config = conf))
img = cv2.resize(img, (h, w))
## Expanding image dims so this represents 1 sample
img = img = np.expand_dims(img, 0)
img = tf.keras.a(chǎn)pplications.resnet50.preprocess_input(img)
extractedFeatures = MyModel.predict(img)
extractedFeatures = np.a(chǎn)rray(extractedFeatures)
DataFrame['flattenPhoto'].a(chǎn)ppend(extractedFeatures.flatten())
### with this all done lets write the iterrrative loop
def ReadAndStoreMyImages(path):
list_ = os.listdir(path)
for mem in list_:
DataFrame['photo_name'].a(chǎn)ppend(mem)
imagePath = path + '/' + mem
LoadDataAndDoEssentials(imagePath, 224, 224)
### lets give the address of our Parent directory and start
path = 'enter your data's path here'
ReadAndStoreMyImages(path)
######################################################
# lets now do clustering #
######################################################
Training_Feature_vector = np.a(chǎn)rray(DataFrame['flattenPhoto'], dtype = 'float64')
from sklearn.cluster import AgglomerativeClustering
kmeans = AgglomerativeClustering(n_clusters = 2)
kmeans.fit(Training_Feature_vector)
A little explanation for the above code:
上面的代碼使用Resnet50(一種經(jīng)過預(yù)先訓(xùn)練的CNN)進(jìn)行特征提取,我們只需移除其頭部或用于預(yù)測(cè)類別的神經(jīng)元的最后一層,然后將圖像輸入到CNN并獲得特征向量作為輸出,實(shí)際上,這是我們的CNN在Resnet50的倒數(shù)第二層學(xué)習(xí)到的所有特征圖的扁平數(shù)組?梢詫⒋溯敵鱿蛄刻峁┙o進(jìn)行圖像聚類的任何聚類算法。讓我向你展示通過這種方法創(chuàng)建的簇。
該可視化的代碼如下## lets make this a dataFrame
import seaborn as sb
import matplotlib.pyplot as plt
dimReducedDataFrame = pd.DataFrame(Training_Feature_vector)
dimReducedDataFrame = dimReducedDataFrame.rename(columns = { 0: 'V1', 1 : 'V2'})
dimReducedDataFrame['Category'] = list (df['Class_of_image'])
plt.figure(figsize = (10, 5))
sb.scatterplot(data = dimReducedDataFrame, x = 'V1', y = 'V2',hue = 'Category')
plt.grid(True)
plt.show()
結(jié)論本文通過解釋如何使用深度學(xué)習(xí)和聚類將視覺上相似的圖像聚在一起形成簇,而無需創(chuàng)建數(shù)據(jù)集并在其上訓(xùn)練CNN。
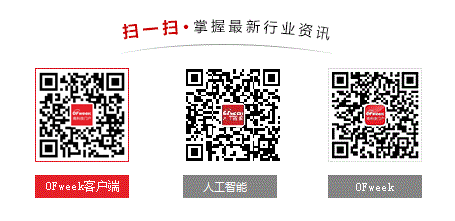
發(fā)表評(píng)論
請(qǐng)輸入評(píng)論內(nèi)容...
請(qǐng)輸入評(píng)論/評(píng)論長(zhǎng)度6~500個(gè)字
最新活動(dòng)更多
-
3月27日立即報(bào)名>> 【工程師系列】汽車電子技術(shù)在線大會(huì)
-
4月30日立即下載>> 【村田汽車】汽車E/E架構(gòu)革新中,新智能座艙挑戰(zhàn)的解決方案
-
5月15-17日立即預(yù)約>> 【線下巡回】2025年STM32峰會(huì)
-
即日-5.15立即報(bào)名>>> 【在線會(huì)議】安森美Hyperlux™ ID系列引領(lǐng)iToF技術(shù)革新
-
5月15日立即下載>> 【白皮書】精確和高效地表征3000V/20A功率器件應(yīng)用指南
-
5月16日立即參評(píng) >> 【評(píng)選啟動(dòng)】維科杯·OFweek 2025(第十屆)人工智能行業(yè)年度評(píng)選
推薦專題
-
10 月之暗面,絕地反擊
- 1 UALink規(guī)范發(fā)布:挑戰(zhàn)英偉達(dá)AI統(tǒng)治的開始
- 2 北電數(shù)智主辦酒仙橋論壇,探索AI產(chǎn)業(yè)發(fā)展新路徑
- 3 “AI寒武紀(jì)”爆發(fā)至今,五類新物種登上歷史舞臺(tái)
- 4 降薪、加班、裁員三重暴擊,“AI四小龍”已折戟兩家
- 5 國(guó)產(chǎn)智駕迎戰(zhàn)特斯拉FSD,AI含量差幾何?
- 6 光計(jì)算迎來商業(yè)化突破,但落地仍需時(shí)間
- 7 東陽(yáng)光:2024年扭虧、一季度凈利大增,液冷疊加具身智能打開成長(zhǎng)空間
- 8 地平線自動(dòng)駕駛方案解讀
- 9 優(yōu)必選:營(yíng)收大增主靠小件,虧損繼續(xù)又逢關(guān)稅,能否乘機(jī)器人東風(fēng)翻身?
- 10 封殺AI“照騙”,“淘寶們”終于不忍了?